Why AI Governance Matters When Implementing AI Tools in Clinical Practices
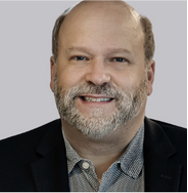

Based on the growing evidence-base and experiences, we can confidently state that diagnostics AI will be an important part of improving healthcare delivery for patients, care teams and the population - and there is a health economic value to be articulated. That being said, uncovering that value and defining a right-sized strategy and governance process for each system and their unique patient, practice and payer dynamics can be difficult. The added dimension of value quantification adds yet another barrier-to-entry for systems and practices struggling in the current environment to just get through each day delivering care. How should a healthcare system even begin to approach this complex challenge of deploying clinical AI?
Recently, this excellent article by my friends at Mass General Brigham (MGB) published in the March edition of the Journal of the American College of Radiology attempts to address this exact challenge. Unless you are truly a diagnostic imaging "nerd," there’s a good chance you may have missed it. The authors of Bernardo Bizzo MD et al. represent a cross-section of the innovation ecosystem at MGB bringing AI into diagnostic medicine – departments of radiology and pathology, the Data Science Office, and medical informatics services. In succinct and clear prose, they outline their successful governance process, solution architecture, and deployment methodology that shepherd commercial and research-grade, diagnostic AI applications from concept/request to clinical deployment and monitoring for lifecycle management. These really are best practices. While it may seem like an overly burdensome process and a large investment of resources, it highlights precisely the type of scrutiny and decision making needed before a health system or physician practice procures, deploys and uses diagnostic AI in patient care. One does not simply start sending images, reports, and lab values to ChatGPT and expect repeatable, robust, and value-add results.
Because of the inherent complexities and potential for harm using AI methods in clinical routine, it is imperative that risk management frameworks and methods be incorporated into the AI governance process. The frameworks and tools used by regulatory bodies may not be the most appropriate to balance the risk vs benefit. We have seen several publications and other discussions in which methods developed by the IMDRF are held forth as a role model for healthcare organization's assessment and evaluation of medical AI. Keep in mind, a health system and the FDA, Health Canada, or SFDA have different missions and must deal with different issues concerning technology deployment. The MGB team does, I think, a nice job describing a "just right" risk assessment mindset. Much more could be written about this topic.
We anticipate some of the usual criticism common when an AMC or large IDN outlines their best practices for adoption of new solutions: not every healthcare institution has Mass General Brigham-scale IT resources, administrative bandwidth, and residents to do the heavy lifting. This is a valid point, but critics need to remember one of the key roles of academic medicine—to translate, evaluate, iterate, and improve new science and technology from promising concept to standard of care. Groups at institutions like MGB, Stanford, Mayo, UCSF, University of Washington, NYU and others have been leading the charge on establishing the scientific, medical, administrative and technical requirements and processes needed to extract value of the (sometimes) astonishing, data-driven technologies that are becoming pervasive both in the zeitgeist and in actual use. It is worth noting that there is also outstanding translational work and best practices being developed and shared by pioneering radiology groups and community practices. An observation from these experiences is that a successful diagnostic imaging AI program requires a multi-disciplinary approach with expertise from IT (infrastructure, applications, and governance), data science, clinical medicine, medical physics, and economics.
We would also like to point out one subtle but important point. Before developing the processes and tools described so eloquently in Bizzo MD et al. there had to be institutional (or at least departmental) buy-in and belief that there is value in pursuing a clinical data science effort. In our experience, many community systems and physician practices have struggled to articulate that value for their own organizations. So, developing a governance process, deployment models and monitoring solutions are moot until a system-specific strategy and roadmap have been established. That is also an existential threat for the host of AI orchestration platforms/marketplaces. It somewhat doesn't matter how many AI-enabled applications you can maybe bring to practice if it is unclear to the buying center if they need any of them. It is further complicated for a busy health system or practice to rationalize and realize true value when nearly every vendor's marketing spiels promise the same things - their AI tools will "maximize outcomes," "seamlessly integrate into your workflow," or "democratize AI." This is why expertise and an ability to articulate healthcare value – in economic terms – is so critical.
At Asher Orion Group we are Activating Medical AI for Improved Outcomes. We fill the gap in resources and expertise that mid-size and community hospitals may not have or be able to allocate in determining a right-sized diagnostic medicine, AI strategy. We provide the additional skills, experiences, and capabilities needed to help translate best practices, like those from MGB, into actions and processes. We work with healthcare systems and provider groups to establish their AI Strategy and Roadmap that aligns to and helps achieve defined outcome objectives while taking into account their current and future IT Systems and Data capabilities.