Insiders Perspective into the FDA’s AI/ML List for the Healthcare Buyers Looking to Activate an AI Strategy
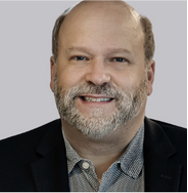

Avoid the AI FOMO Hype
If you have even peripheral involvement or interest in medical AI, you invariably have seen in various organization social feeds, presentations or independent assessments some reference to the information provided by the US FDA that lists market-cleared, medical devices incorporating AI/ML technologies: https://www.fda.gov/medical-devices/software-medical-device-samd/artificial-intelligence-and-machine-learning-aiml-enabled-medical-devices. It is a great resource and a "one-stop-shop" to view productization activity and see trends in product capabilities. It is also a means for gaining insight into the agency's evolving frameworks for regulating Medical Device Software that make heavy use of modern, data-driven technologies like deep learning.
The list was recently updated on October 5 with clearances granted the past 2 months. That update has also spurned countless power point deck items claiming, "Hundreds of AI products are now on the market!" We have seen statistics quoting all 521 devices listed on the list as proof that the regulated healthcare market is teeming with adoption of AI to generate “Fear Of Missing Out” (FOMO) for investors ("invest in my medical imaging AI company or platform NOW!") or provider/physician customers ("you need to buy our AI app/platform/services before it's too late!"). We have also seen the list used as bragging rights by major manufacturers to underscore how innovative and “on the edge" they are because of the number of cleared products in an "ours goes to 11" type of marketing logic. We want to help give some perspective and insight to this list to help educate and inform the community to use the data in a more refined fashion.
For those who have been involved in radiology/imaging for a while, you may recall in the early 2000s when multi-slice CT became a reality for the broad market. This incredible technology did enable (and still does) big advances in diagnostic capabilities. At the Radiological Society of North America meetings in 2004 and 2005, scanner vendors went nuts in promoting "we have more slices (or detectors) than competitor X or Y," At the Radiological Society of North America meetings in 2004 and 2005, scanner vendors went nuts in promoting "we have more slices (or detectors) than competitor X or Y," or "they claim 64 but really they have 2, 32-slice rings," or "our rotation time is .2 seconds better than Y." These boasts did not help customers develop an appreciation of the clinical value of the technology. We are wary that at this year's RSNA we may see a flashback to the early 2000's with vendors plastering their booths with "we have 24.5% more AI than our competitors" or "more AI than anyone else," etc. My goal here is to put the listing in context and to allow the reader to more critically assess boasts and posts by manufacturers claiming their AI superiority. To get there, though, we need to visit a few topics. First, let us define a working definition of “AI in Healthcare,” then we will talk about the technology underpinnings of medical devices, and finally we will discuss a few critical aspects of the regulatory process for medical devices in the US.
AI in Healthcare
There is no single, good definition of an “AI in healthcare.” Nor is there any easy, agreed-upon definition of Artificial Intelligence. John McCarthy, the famous Stanford Computer Scientist and creator of Lisp, made a definition we like. He wrote in 2004, “[Artificial Intelligence] is the science and engineering of making intelligent machines, especially intelligent computer programs.” ML and data science innovations enable “intelligent” behaviors of systems and devices in different ways in many aspects of our day-to-day lives. The ML may be overtly evident as in auto-pilot and self-guidance modes in cars. An example of the less obvious ML algorithms are those embedded in our email software parsing and analyzing our grammar. At a minimum, an intelligent machine should be able to process input, make some decision, and act or recommend some action based on the decision process. In healthcare applications, ML technologies are and will be implemented in various ways, and we will examine some of the manifestations later.
Most of the regulated radiology, cardiology, dermatology, and ophthalmology products cleared by the FDA use supervised machine learning methods. This is a paradigm in which an algorithm is ‘taught’ by looking at MANY examples of something given a label by us, the human masters. The advanced mathematics of the ML algorithms develop a way to - on its own - detect features in, classify, or characterize the input data. We say that the computer code develops a ‘model’ of the real world. That model accepts inputs (e.g.: medical images), processes those data, and returns an output. Output information could be a label that identifies the input images as a cat, hot dog, Renal Cell Carcinoma, or could be a ‘flag’ indicating that the study should be prioritized by the radiologist because the ML algorithm detected something abnormal.
We contend that the most transformative and powerful applications of AI in healthcare are those in which computer systems gather information from multiple sources, analyze it with computationally creative means, and deliver some recommendation or guidance to the human user in an ‘intelligent’ fashion. The processing and analysis of raw or ‘source’ data (images, signals, lab values, physician notes) will usually need to occur somewhere removed from the data source, especially if the data source are medical device systems like MRI or CT/PET scanners. This is because: a) oncologists, neurologists, and other clinicians are not going to be near or at the device; b) complicated diagnostic decisions typically require more data than just what a scanner or patient monitor produces; and c) the information and results of the intelligent decision making will be made in the information systems where the physicians do their ‘knowledge’ work (like an Electronic Health Record system or a PACS for radiologist).
To better appreciate the state of “AI” in medical products, one should stratify the applications and use cases by the amount and type of intelligent behaviors exhibited by the solution. The potential power of machine intelligence for assisting in making more precise and faster diagnostic and therapeutic decisions by analyzing multiple data streams and automating tasks in the radiologist or clinician workflow should be considered differently from ML technologies denoising or filtering data processed within a medical device system. This distinction is important to truly comprehend the current AI market.
Medical Device Technology
Medical devices like CT scanners and ECG monitors perform a lot of digital signal and image processing on the raw sensor data collected from a patient to make images or waveforms that are interpretable by a human physician. In CT scanners those sensor values are X-Ray energy levels and in ECG monitors the sensors collect small voltages from electrodes on the patient’s skin. As the sensors and acquisition systems have become more advanced there has been a need to speed up and improve the computational abilities in the devices. There are many applications for ML techniques in improving these processes. An example might be the use of supervised, Deep Learning ML to filter out noise and artifacts from CT, MRI or ECG data. Another example of ML in this stage of the device processing is identifying organs or other structures and highlighting or “segmenting” them. In this case, when the human cardiologist (using ultrasound to diagnose a heart condition) sees the images on the scanner, the mitral valve is highlighted along with its trajectory of movement. Because Deep Learning and other advanced ML techniques have great utility in filtering noisy images and in helping reduce the time to generate medical images from the raw sensor data, it is not surprising that Deep Learning technologies are incorporated into many of the image and signal processing pipelines within MRI, CT, and Ultrasound imaging systems.
Please, don’t think we are discounting the innovation and significance of these very important capabilities that inform medical decisions and make devices and systems easier to use while reducing the burden on the healthcare provider operator. An example of this valuable automation is in the cardiac ultrasound scenario in which an ML algorithm delineates and measures the mitral valve or the left ventricle so the cardiologist can focus on the clinical picture rather than clicking mouse buttons and keyboard tapping. The ML algorithms streamline and automate some of those steps and can provide more consistent measurements of structures. These functions, however, are not automating nor guiding a complex diagnostic decision.

Medical imaging as a discipline has been enabled by breakthroughs in computational and numerical methods since the invention of CT, MRI, ultrasound, and nuclear medicine imaging modalities. One such critical innovation was the development of the Fast Fourier Transform algorithm that, when implemented in custom or generalized microprocessors, made possible the routine processing of data from MRI and CT scanners within a timescale feasible for patient care. We don’t believe this type of enabling – yet powerful and awesome – computing technology and use-cases match what we mostly think of when we consider the potentially transformative power of “AI” to healthcare.
Understand the FDA Data like an Expert
When reviewing the list of FDA-cleared, AI/ML medical device products, one should not naively just filter by medical specialty (like Radiology or Cardiology) and manufacturer and count the number of products and pronounce “we’ve got the most AI!” We present here an interpretation of the raw, FDA data that might help you gain an ‘expert’ view of the emerging landscape.
A fuller description of the process for bringing a medical device product to market – especially Medical Device Software – deserves its own article. A few points, though, are critical for contextualizing claims of "who’s got the most AI.” Most imaging-based AI products are considered, in the language of regulated products, to pose “medium risk” to users and patients and thus go to market following a process known as a 510(k)-pre-market clearance path (note: clearance is not the same as approval... again, a whole other article). Market clearance from the FDA gives a manufacturer permission to market their medical device with specific performance claims and functions that the FDA agrees are reasonable and that match those of a similar device that is already on the market.
The FDA uses 3-letter codes to categorize types of medical devices into groups having similar form and function. These product codes are really important when considering the list of ~500 devices in the AI/ML listings. When you look at products in the AI/ML list, please note the product code classification. If the AI/ML product has categorization code of a "device” like JAK for CT Scanners, it’s highly likely that the AI “Product” is performing functions within the medical device system, such as filtering, reconstructing the image, or automating measurements. While these are important capabilities, they are not typically “intelligent decision making” software. So, you should carefully review AI regulated products whose product classification codes indicate that it is a core component or extension of an imaging system; example product codes: IYN= System, Imaging Pulsed Doppler, Ultrasound; JAK = System, X-Ray, Tomography, Computed; or LNH= System, Nuclear Magnetic Resonance Imaging. The AI/ML inside these systems allows the devices to perform great diagnostic tasks, but AI/ML methods are an enabling technology much like the FFT in the 1980 and 1990s.
The AI Product Landscape
What are product types that might be more representative of an “intelligent system?” A clue to how the FDA classifies level of capability is gained by parsing the name of product classifications that do perform tasks more naturally consider to be intelligent. Product types starting with the phrase “Computer Aided XX” were created and are being expanded by FDA to describe software products, using ML or not, that perform computational activities to augment or automate cognitive tasks usually done by a physician.
There are several newer product classification codes from the FDA for software products that align to our definition of an impactful, healthcare AI solution. Examples are software that automatically analyzes medical images and flags the study for high priority reading, software applications trained to distinguish potentially malignant tumor from benign tumors, or an application that analyzes a patient’s medical imaging study and potentially hundreds of other clinical data to develop a probability of a rare disease. A full accounting of the product classification codes for automated and standalone AI/ML software applications is beyond the scope of this article. You can, however, find the definitions of the product codes and the "special controls” the FDA expects a manufacturer to employ when building, testing and deploying their product at: Product Code Classification Database | FDA. The product types we think to be most representative of a “medical AI” application as contemplated in this article are: MYN, NFJ, OEB, POK, QAS, QBS, QDQ, QFM, QIH, QJU, and QKB. Let’s review 2 of these product classes.
What used to be denoted a Computer Aided Detection device by the FDA up until 2019 is now called a Medical Image Analyzer or "CADe" product and has the product code MYN:
An MYN is: “intended to identify, mark, highlight, or in any other manner direct the clinicians' attention to portions of a radiology image that may reveal abnormalities during interpretation of patient radiology images by the clinicians. This device incorporates pattern recognition and data analysis capabilities and operates on previously acquired medical images. This device is not intended to replace the review by a qualified radiologist, and is not intended to be used for triage, or to recommend diagnosis."
A second product type in the new “AI” is called a Radiological Computer-Assisted Prioritization Software For Lesions and has the product code QFM. It is further defined by FDA as: “The device provides triage or notification that is informed by machine learning, artificial intelligence, or other image analysis algorithms. This type of device establishes effective triage within a specialist's queue based on high sensitivity and specificity >95% AUC.”
Of importance in this definition is the clause “provides triage or notification;” these software products are making some type of judgement based upon automated analysis of an imaging study. However, the notification message and information to the physician cannot consist of “Hey! Look at this study because I think they are going to have a heart attack!!” The added judgements and decisions required for an analytic to arrive at a determination that a patient is at risk of a heart attack makes that product a different type of software product, namely a Computer Aided Diagnosis product. It will have its own product code, different types of constraints, and require different evidence to convince the FDA that the algorithm is safe and efficacious.
Refined Analysis of FDA AI Products List
After excluding product codes for modality devices, medical imaging and management systems, and non-radiological devices, and then only considering the product codes listed in the previous paragraph (MYN, QFM), the resulting list of products and vendors is very different from that which you may have seen posted and commented upon by analysts and large manufacturers.
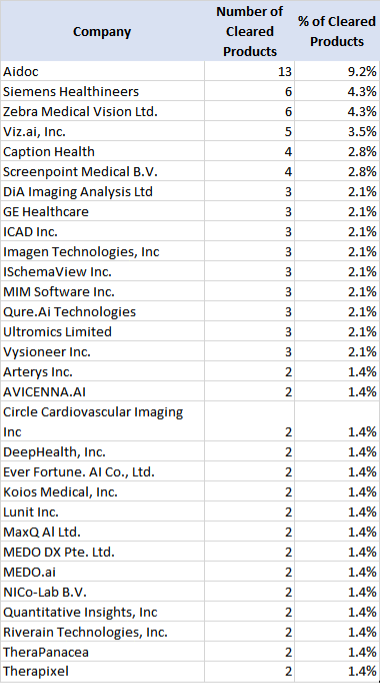
The list is not dominated by large MedTech vendors or scanner OEMS, as we often refer to GE, Siemens Healthineers, Philips, Canon, and United. Siemens Healthineers is the notable exception, and we will discuss shortly. But the key takeaways are: a) the volume and number of “true” imaging AI products is far less than the 550+ products often quoted; b) AI ISVs (independent software vendors) are developing and selling the newer and riskier AI products; and c) the distribution has a long tail with many vendors having 1 or 2 products. Table 1 excludes vendors with only 1 “AI” product cleared up until October of 2022.
You can also infer the different strategies being taken by the imaging MedTech OEMs. Whereas GE Healthcare tops the “total” list of AI/ML products, the majority of their product types are tightly coupled into or with acquisition systems. Whereas Siemens Healthineers also has a lot of other AI/ML products in the master list, they rank high on my list because of their strategy with the AI-Rad Companion solution set. The Rad Companion software products are architected to process the imagery "off board" from the Siemens Healthineers scanner so they can process and interpret other vendors’ imagery, with the results being incorporated into various PACS or other radiologic workflows. GE, PHILIPS, and Canon seemingly are investing in their own AI/ML methods to differentiate acquisition and generation processes and partner with AI ISV companies bringing the “clinical” AI functionality as described above. This is not a new or different trend -- it has been the norm in the imaging industry for 20+ years. Many independent, visualization and computational products have been “bundled” into larger manufacturer deals, such as iCAD, MergeCAD, TeraRecon products, etc.
Takeaways
As a busy healthcare provider, investor, or healthcare innovator, gaining insights to the impact of new, complex technologies like Artificial Intelligence to the medical device market can be difficult. The FDA developed and made accessible this valuable informational resource – the ““Artificial Intelligence and Machine Learning (AI/ML)-Enabled Medical Devices” to demonstrate the diversity and velocity of innovation in Medical Device Software. This resource is a summary of decisions for medical device products that incorporate or make use of computational technologies that can be categorized under the broad umbrella of “Artificial Intelligence”. It is important to appreciate the nuances of the FDA’s, regulatory process, and the nature of software medical products, however, before making direct conclusions from the data. In other words, the data resource should not be a primary source of market and competitive insights.
At Asher Orion Group, we have years of medical device development experience and knowledge of the market learned from conceiving, designing, bringing to market, and supporting the sale of analytical/ML, medical device software. We use that experience to develop an informed, Point-Of-View (POV) about the landscape of regulated, AI/ML products and are happy to present this analysis.
Critical to developing this informed, POV is first defining and clarifying the types of “artificial intelligence” as applied to medical devices and medical device software. Realize that for any product to be on the FDA list, it must either be part of a medical device system, or functions as a standalone device as defined by the US FD&C* Act. While there is a LOT of value and impact by incorporating AI/ML methods within medical devices, we posit that the broader, transformational potential of AI to medicine is best realized by products that make diagnostic decisions, assist physicians in making decisions, or that actuate a treatment or intervention based upon their abilities to derive insights from data. We, therefore, filtered and analyzed the ‘raw’ FDA listing with that distinction in mind.
When assessing healthcare technology value, in general, quantity shouldn’t trump quality. Numerical rankings can be insightful, though, to build a more-informed picture of the innovation landscape. Informed by the criteria above, our analysis shows that the two vendors with the most cleared, AI/ML products that directly inform licensed medical professionals in the interpretation and processing of diagnostic, medical imaging are Aidoc and Siemens Healthineers.
Aidoc’s product strategy has been to focus on assisting the radiologist in prioritizing their work and to be reminded if a potentially, urgent finding may have been missed in their read. “There is no shortage of healthcare AI options, but significant gaps exist among them. You can have the best algorithm in the world, but if you can’t deliver data in a streamlined and actionable way, it won’t be effective,” said Elad Walach, co-founder and CEO of Aidoc. “The value of AI is unleashed through a strategic, scalable, and intelligent unified platform that empowers physician decision-making through real-time data. Our approach to innovation has married our AI engineering ability with real-world clinical requirements to develop a solution that provides actual and immediate value to both health systems and patients.”
Siemens Healthineers’ focus has been largely towards using the discriminatory and detection power of DL/ML methods in detecting and/or characterizing findings that may be secondary to the radiologist’s primary mission in reading a study. “One of the keys to our success in Artificial Intelligence is our focus on high-quality clinical data for algorithm development. Siemens Healthineers has built out specialized diagnostic reading teams that provide analytical expertise on a database of over 1.2 billion curated images, clinical data and specialized reports to assist in training the AI algorithms,” said Peter Shen, head of digital health for North America, Siemens Healthineers.
We hope this article is useful to the overburdened buyers in the imaging community either trying to understand the AI/ML landscape or whom are in the process of developing an activation strategy for their practice or health system. Asher Orion Group is happy to further this discussion and analysis with those buyers. Our goals are to help narrow-down the landscape, assess AI solutions relevant to your needs, assist in the optimal configuration of those solutions, and to monitor the performance and ROI of AI/ML innovations regardless of the manufacturer, integration and run-time platform, or IT deployment model. AOG – your trusted partner to help activate Medical AI outcomes.
*Federal Food, Drug and Cosmetic Act:21 US CFR Chapter 9